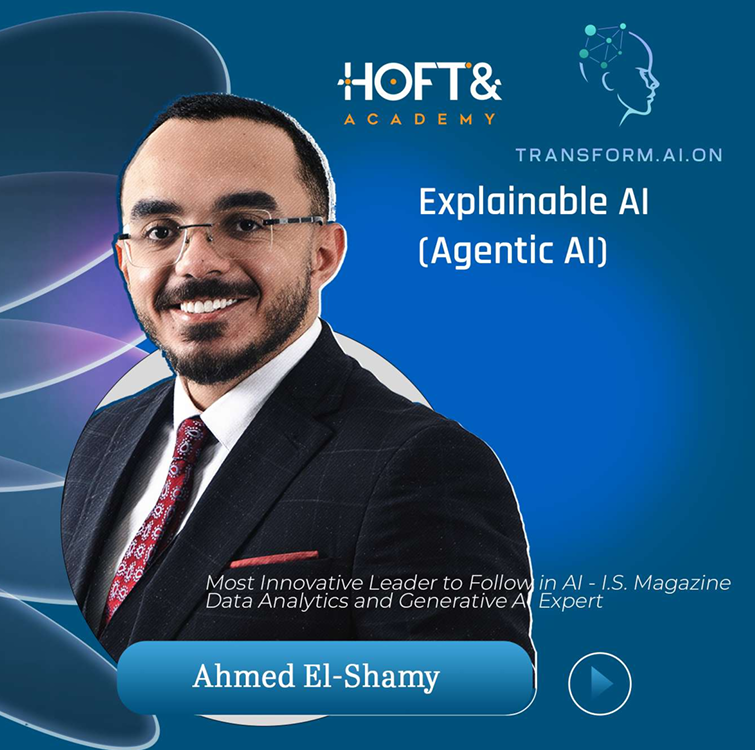
Explainable AI in Finance: Building Trust Through Transparency in Financial Decision-Making | transform.ai.on Weekly Series
🔷 AI Weekly Series – Post #5 🔷
Unlocking AI Transparency in Finance! 🚀
AI models often act as ‘black boxes,’ making predictions hard to understand, leading to compliance risks.
✨ Enter Explainable AI (XAI)! ✨
XAI makes AI transparent and interpretable, ensuring trust in financial decisions.
⚠️ The Risk:
Most AI models prioritize performance over clarity, leaving users in the dark.
🔍 Why It Matters:
XAI helps you see how AI makes decisions, reducing risks and improving automation reliability.
💡 Stay ahead with expert insights from Ahmed El-Shamy 🥇
In today’s data-driven financial landscape, artificial intelligence has become an indispensable tool for institutions seeking competitive advantage. However, as AI systems grow increasingly sophisticated, they often become less transparent—creating significant challenges for organizations that must justify their automated decisions to regulators, stakeholders, and customers.
The Black Box Problem in Financial AI
Financial institutions increasingly rely on advanced AI models to drive critical functions from credit decisioning to fraud detection. Yet many of these systems operate as “black boxes,” producing predictions with little visibility into their reasoning process. This opacity creates substantial challenges:
- Regulatory compliance becomes difficult when decisions cannot be explained
- Customer trust erodes when financial outcomes seem arbitrary
- Risk management suffers without clear understanding of model behavior
- Ethical concerns arise when biases cannot be identified and addressed
“When billions of dollars, regulatory compliance, and economic stability are on the line, stakeholders cannot afford opaque AI models that lack interpretability,” notes financial technology experts4.
What is Explainable AI?
Explainable AI (XAI) refers to artificial intelligence systems designed to make their decision-making processes transparent and understandable to humans. Unlike traditional black-box models, XAI provides visibility into how and why decisions are made, allowing financial institutions to:
- Understand the rationale behind AI-driven predictions
- Provide clear, documented explanations to regulators
- Build trust with customers through transparent decision-making
- Identify and address potential biases or errors in model outputs
As one industry analysis puts it: “Explainable AI ensures that financial institutions can trust and validate model outputs, reducing the likelihood of errors, biases, or unethical outcomes in sensitive applications like credit scoring, fraud detection, and risk assessment”1.
Why Financial Institutions Need XAI Now
Regulatory Pressure
Financial regulations worldwide increasingly demand transparency in automated decision-making. From the EU’s GDPR and upcoming AI Act to various financial oversight frameworks, institutions must be able to explain their AI-driven decisions or face potential penalties48.
“Financial institutions operate in one of the most heavily regulated industries worldwide, and these regulations increasingly demand openness in automated decision-making processes,” notes the Corporate Finance Institute8.
Trust Building
AI-powered decision-making is only as valuable as the trust it inspires. Without transparency, customers, investors, and financial managers question whether AI recommendations align with their goals and risk tolerance. XAI provides clear reasoning that fosters confidence in automated systems4.
Risk Mitigation
Blindly following AI models exposes institutions to potentially catastrophic financial risks. XAI helps financial professionals vet AI-driven decisions, flag potential biases, and prevent costly errors before they escalate49.
Enhanced Decision Support
Beyond compliance and risk management, XAI empowers financial professionals by offering insights into AI models’ decision-making processes. This allows experts to refine strategies, optimize algorithms, and improve overall performance with a data-driven yet fully explainable approach4.
Key Applications of XAI in Finance
Credit Decisioning
For loan approvals, XAI brings clarity to credit decisions. Rather than simply accepting or rejecting applications based on opaque AI outputs, financial institutions can now provide clear explanations for their lending choices. When a loan is denied, the system can identify specific factors like debt-to-income ratios or payment history that influenced the decision5.
Fraud Detection
In fraud detection, XAI enables investigators to understand why certain transactions are flagged as suspicious. This allows fraud teams to pinpoint patterns and anomalies that trigger alerts, improving both detection accuracy and investigation efficiency5.
Risk Management
Financial institutions can now trace how AI models assess market risks, evaluate investment portfolios, and forecast potential threats. This transparency is crucial for regulatory compliance, as authorities increasingly demand explanations for AI-driven risk assessments59.
ESG Assessment
Explainable AI is also being adopted to assess Environmental, Social, and Governance (ESG) risks, a growing area of focus for financial institutions. XAI helps explain how various sustainability factors influence investment decisions and risk profiles11.
Implementing XAI: Practical Approaches
Breaking Down Decisions
One effective XAI approach involves breaking the decision-making process into discrete elements. Instead of analyzing a case holistically, a model evaluates individual parameters before transferring outputs to a transparent, rule-based model1.
For example, in credit application processing, an explainable model would evaluate annual income, outstanding debt, and employment stability separately. The reasoning becomes traceable and justifiable—instead of a vague refusal, the decision-maker can point to specific factors1.
Statistical Tools
Several statistical techniques enhance explainability while maintaining model performance:
- SHAP (Shapley Additive Explanations): Quantifies each feature’s contribution to a prediction
- LIME (Local Interpretable Model-agnostic Explanations): Creates simplified explanations of complex models
- Monotonicity constraints: Ensure that relationships between variables follow logical patterns
These approaches help maintain model robustness while improving transparency1410.
Accelerated Computing
Recent innovations have made XAI more practical for large-scale applications. GPU-accelerated computing can now generate explainability profiles for entire credit portfolios in minutes rather than days, making XAI commercially viable at scale10.
The Future of XAI in Finance
As AI continues to transform financial services, explainability will become increasingly critical. The financial institutions that thrive will be those that successfully balance the power of advanced AI with the transparency demanded by regulators, customers, and stakeholders.
“Explainable AI is no longer a luxury but a necessity for regulatory compliance, risk mitigation, and fostering trust,” according to recent industry analysis9. As we move toward more sophisticated AI systems, including generative AI applications in finance, the need for robust explainability frameworks will only grow.
By embracing XAI now, financial institutions can build more trustworthy systems, reduce regulatory risks, enhance decision quality, and ultimately deliver better outcomes for their customers and shareholders.
Conclusion
In an era where financial decisions increasingly rely on artificial intelligence, explainability has become essential. XAI bridges the gap between powerful AI capabilities and the transparency required in regulated financial environments. By making AI decisions understandable, financial institutions can harness the full potential of these technologies while maintaining the trust and accountability that form the foundation of the financial system.
As we look toward a future where AI plays an ever-expanding role in finance, explainability will be the key that unlocks AI’s full potential while ensuring it serves human needs responsibly and ethically.
Tag:AI, Finance, transform.ai.on