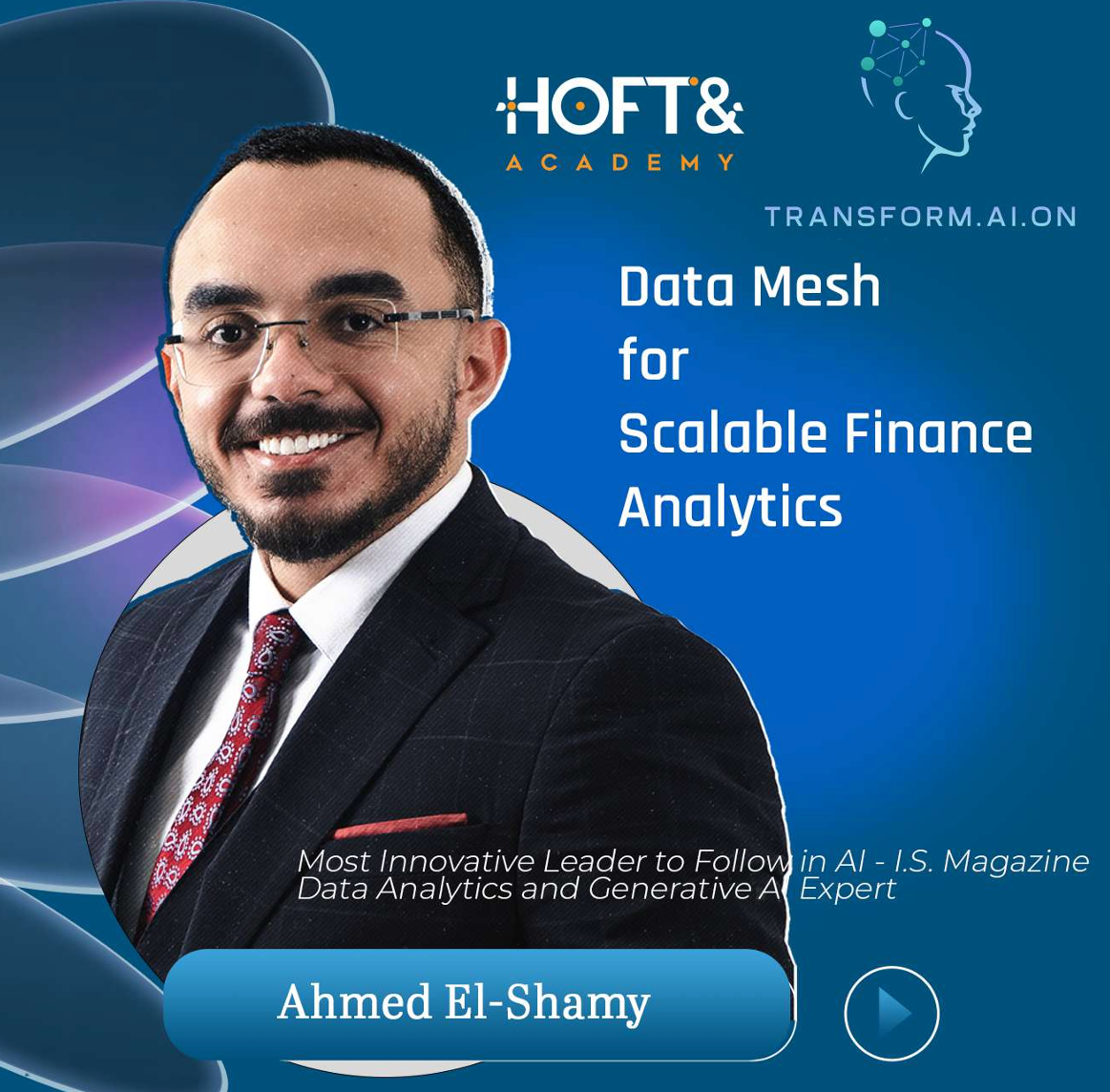
How Data Mesh is Transforming Finance Analytics for Scalability and Speed | transform.ai.on Weekly Series
Empowering domain-driven data ownership for faster insights in financial services
🔷 AI Weekly Series – Post #3 🔷
Traditional data systems slow down decision-making. Data Mesh changes that by giving teams control over their data for faster, smarter insights! 🚀
Are you ready to make your finance analytics scalable? 💡📊
Stay tuned for more expert insights with Ahmed El-Shamy 🥇
How Data Mesh is Transforming Finance Analytics for Scalability and Speed
In today’s fast-moving financial sector, data bottlenecks can break decision-making. Traditional data architectures—centralized, siloed, and rigid—are failing to keep up with real-time demands.
Enter: Data Mesh — a decentralized approach that empowers domain teams to own their data and operate at speed.
In this blog, we’ll explore how Data Mesh is revolutionizing finance analytics, making them scalable, agile, and smarter than ever before.
What Is Data Mesh and Why It Matters in Finance
At its core, Data Mesh is a modern data architecture that shifts from centralized data ownership to domain-driven responsibility. Rather than routing everything through a central IT team, Data Mesh allows individual departments—like risk, lending, or compliance—to manage and serve their own data as products.
Why Finance Needs It:
- High volume, high-speed data streams
- Regulatory compliance demands
- Fragmented data across systems (CRM, ERP, trading platforms)
- Slow decision-making due to central bottlenecks
In a Data Mesh model, finance teams get real-time access to the data they need, when they need it, without waiting on another department.
Key Benefits of Data Mesh for Scalable Finance Analytics
1. Domain-Driven Ownership = Better Data Quality
When domain teams own their data, they ensure its accuracy, timeliness, and usability—key traits needed in high-stakes environments like banking.
🧠 Think of each department as a mini data company within your organization.
2. Faster Time-to-Insight
Instead of waiting weeks for centralized teams to prepare reports, domain experts can query their own validated datasets directly, accelerating real-time analytics.
3. Scalability Without Complexity
Adding new data sources or analytical models becomes easier since each domain is independently responsible, yet federated under shared standards.
4. Built-In Compliance and Governance
With federated governance, global standards exist (e.g., GDPR, PCI-DSS), but each domain implements controls locally—offering regulatory alignment with agility.
Use Cases: How Financial Institutions Apply Data Mesh
Retail Banking:
Customer data products managed by customer service and digital banking teams allow personalized loan offerings and predictive churn analysis.
Risk & Compliance:
Risk modeling teams manage their own datasets for stress testing, fraud detection, and compliance analytics without relying on legacy reporting chains.
Capital Markets:
Real-time trade data products enable microsecond-level decisions with localized ownership and performance tuning.
Implementation Strategy for Data Mesh in Financial Services
1. Identify Business Domains
Map your org by lines of business or functions: lending, credit, AML, etc.
2. Define Data Products
Each domain defines what “products” they’ll offer—e.g., customer_transactions
, risk_exposures
, etc.
3. Establish Federated Governance
Global policies (security, access control) combined with local autonomy for how data is served and consumed.
4. Build Self-Serve Infrastructure
Invest in tools that enable:
- Data discovery
- Version control
- Data lineage
- API-based access
Recommended Stack: Snowflake, dbt, Monte Carlo, Apache Kafka, Databricks
Challenges to Expect (And How to Overcome Them)
🧱 Cultural Resistance
Shifting data ownership requires retraining and buy-in across teams. Start with pilot programs to prove value.
🧱 Skills Gaps
Domain teams may lack data engineering knowledge. Invest in training programs and enablement.
🧱 Legacy System Integration
You don’t need to rip and replace—layer Data Mesh gradually, integrating with existing lakes and warehouses.
Final Thoughts: Is Your Finance Team Ready for Data Mesh?
Data Mesh isn’t just a trend—it’s a fundamental shift. For financial institutions aiming to stay competitive, it delivers faster decisions, higher data trust, and scalable innovation.
By decentralizing data ownership, empowering domain teams, and ensuring compliance through federated governance, Data Mesh transforms finance analytics from a bottleneck into a business accelerator.
💬 Ready to start your journey? Let’s talk implementation strategy tailored to your finance team.
✅ SEO Optimized FAQ
What is Data Mesh in financial services?
Data Mesh is a decentralized data architecture where domain teams in financial institutions own and manage their data products, enabling faster insights and greater agility.
How does Data Mesh improve finance analytics?
It empowers business units to directly manage their data, ensuring higher quality, real-time access, and better decision-making.
What are the challenges of adopting Data Mesh in banks?
The biggest hurdles include cultural resistance, skill shortages, and integrating legacy systems—but all can be overcome with proper planning.
How is Data Mesh different from a data lake or data warehouse?
Unlike lakes or warehouses, Data Mesh distributes responsibility across business domains, treating data as a product rather than a centralized asset.
Tag:AI, Data Analytics, Finance, transform.ai.on